Researchers from the REGALE project published a paper in Nature Scientific Data and the first-of-its-kind open dataset for HPC telemetry. The paper with the title “M100 ExaData: a data collection campaign on the CINECA’s Marconi100 Tier-0 supercomputer” summarizes the results of a research project that started almost ten years ago at the University of Bologna in cooperation with the largest Italian computing centre CINECA.
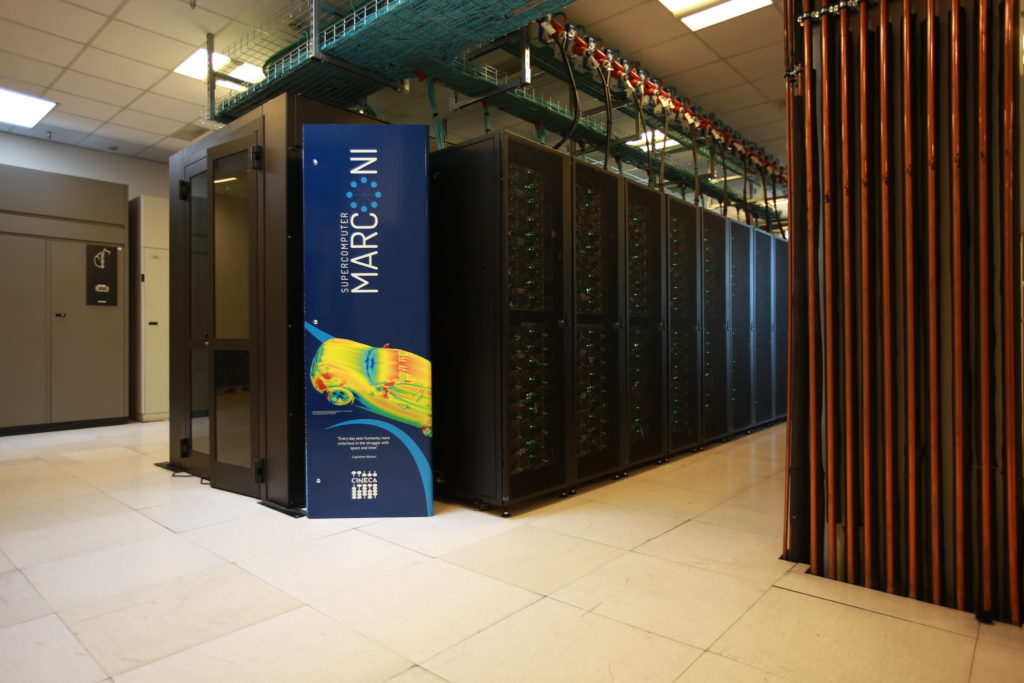
Andrea Bartolini, associate professor at the Department of Electrical, Electronic, and Information Engineering at the University of Bologna, played a key role in the project and answered some questions for us.
Can you tell us more about your research and what is special about the M100 ExaData?
I’ve been working at the University of Bologna for quite a while, and I’ve been collaborating for many years with CINECA. My focus is primarily on improving the energy efficiency in HPC centres. In 2014 we started to build a new monitoring framework for HPC systems. Our goal was to monitor all the processes in the data center and to optimize them. Our research team designed the monitoring framework EXAMON that we later deployed at Marconi100, a tier-0 supercomputer at CINECA. This dataset unveils a holistic view of the supercomputer, encompassing management, workload, facility, and infrastructure data gathered over two and a half years of operation. The dataset, which is available via Zenodo, is the largest ever made public, with a size of 49.9TB before compression.
That sounds impressive, but why is this research important?
It’s important for many aspects. One of them is to improve availability. Because at the end of the day, with CINECA’s HPC resources we are offering a public service. If we increase the quality and the availability of this service, the value of the investment increases and also the outcome in terms of science produced. Another goal is to add automation. High performance systems are very complex. Even operating at their own optimal efficiency, it’s not so simple. By adding automation to that, we try to reach a higher efficiency point. Then of course you have energy and power consumption, translating directly into costs which we want to decrease. Another aspect is to have a good overview of the system. We need to understand how the system behaves, in order to improve future technologies.
How does this research relate to the REGALE project?
Our research is partially funded by the EuroHPC Joint Undertaking through the REGALE project. Within REGALE we are studying how we can optimize the cooling system to make it more energy efficient. Furthermore, we work on strategies for power capping. We do that from two sides. One side is what we call “sophistication” in REGALE. This is about writing new algorithms that we need for power capping. The other side is having a common tool set which is interoperable and allows to implement the sophistication in production in a system.
What else can the data be used for?
My team and I already use the data in several works, for example for anomaly detection and prediction. Moreover, we have a chain of research where we use the data for training models. With this large dataset we plan to predict when a node will be malfunctioning and other types of predictions like job power consumption. These are just a few ways to use the data. By releasing the dataset to the communities, we hope that the researchers will find additional use cases. That is the reason why we put so much effort in preparing the dataset. We spend six months preparing the raw data for publication. We wanted to do as little pre-processing as possible but we had to clean out redundant information, anonymize the data and so on. I think we now reached a good level of compression and we are excited to see future research based on this dataset.
Scientific Paper:
https://www.nature.com/articles/s41597-023-02174-3
Source Code and Links to the data sets: